Harnessing the Power of Large Language Models for Multimodal AI
- July 26, 2024
- Posted by: Anand Borad
- Category: Artificial Intelligence
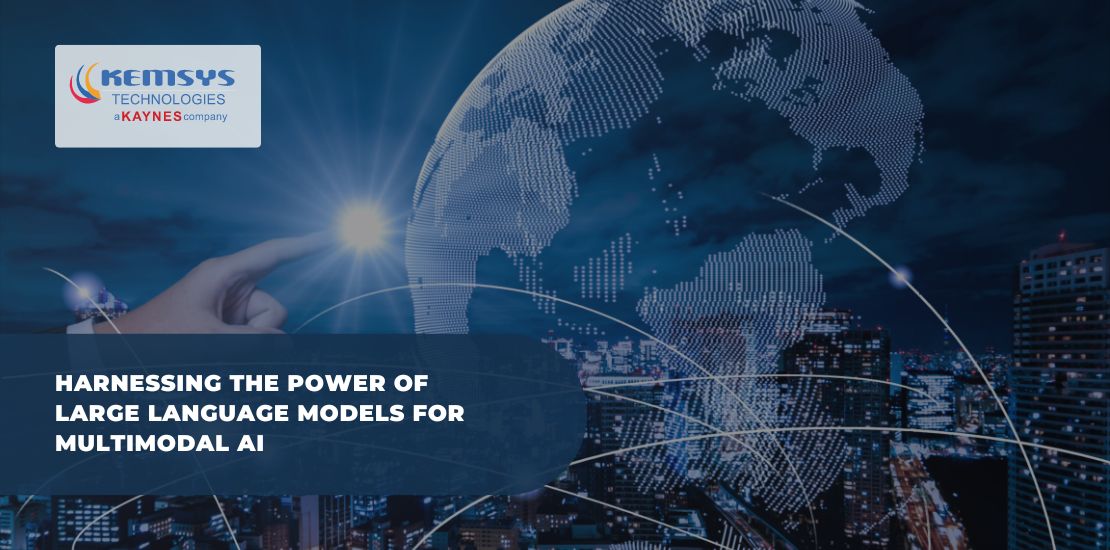
We are now seeing AI assistants not only respond to your text queries but also understand and analyze images, videos, and even your tone of voice. This is the power of multimodal applications, which combine multiple forms of data to create a richer, more intuitive user experience.
Large Language Models (LLMs) like GPT-4 are at the heart of this technological revolution, which has demonstrated remarkable abilities in understanding and generating human-like responses. When integrated with other AI models, LLMs can give birth to new possibilities, transforming industries from healthcare to entertainment.
According to a recent report by Gartner, the market for multimodal AI applications is expected to grow significantly, with a projected CAGR of 25% over the next five years.
This growth is driven by increasing demand for more sophisticated AI solutions that can provide deeper insights and more personalized experiences across various industry verticals. As companies strive to stay competitive, the integration of LLMs in multimodal applications will be a key driver of innovation, efficiency, and customer satisfaction.
Understanding Multimodal Applications
Definition
Multimodal applications are systems that process and understand information from multiple modalities, such as text, images, audio, and video.
Examples include virtual assistants that can understand voice commands and respond with text or speech, healthcare diagnostic tools that analyze both medical images and patient records, and educational platforms that incorporate text, visual aids, and interactive elements.
Importance of Integrating Multiple Data Types
Integrating multiple data types allows AI systems to create a more holistic understanding of complex situations. For instance, in healthcare, a diagnostic tool that can analyze both medical images and textual patient records to assist in providing more accurate diagnoses. In customer service, a virtual assistant that understands text, speech, and visual cues can offer a more seamless and intuitive user experience.
Challenges and Opportunities
While integrating multiple data types presents opportunities for enhanced AI capabilities, it comes with its own challenges. These include the need for large, diverse datasets to train multimodal models, the complexity of combining different types of neural networks and ensuring the seamless interaction between different data modalities. However, overcoming these challenges can lead to significant advancements in AI, enabling more comprehensive and context-aware applications.
Related Content: Artificial Intelligence Use Cases in Industrial Manufacturing
The Role of LLMs in Multimodal Applications
Processing and Understanding Textual Information
LLMs excel at processing and understanding textual information, making them a valuable component in multimodal applications. They can generate human-like responses, summarize long documents, and provide context-aware information, enhancing the overall performance of multimodal systems.
Integration with Other AI Models
LLMs can be combined with other AI models, such as image recognition models and speech-to-text models, to create powerful multimodal systems.
For example, a virtual assistant might use an LLM to understand and generate text, while relying on image recognition models to interpret visual data and speech-to-text models to process audio inputs.
Benefits of Using LLMs in Multimodal Contexts
Using LLMs in multimodal applications offers several benefits, including enhanced understanding and improved user experiences.
LLMs can provide context-aware responses by considering information from multiple modalities, leading to more accurate and relevant interactions.
Additionally, their ability to generate human-like text can make interactions with AI systems feel more natural and intuitive.
Key Technologies and Architectures
Enabling Architectures
The development of multimodal applications relies on advanced architectures such as transformers and attention mechanisms. These architectures enable the efficient processing of large datasets and the integration of different types of data.
For instance, transformers, which form the backbone of LLMs, can be adapted to process multimodal inputs by incorporating additional layers for handling images or audio.
Integration of LLMs with Other Models
Integrating LLMs with other models involves combining their strengths to create more powerful and versatile systems. For example, combining GPT-4 with convolutional neural networks (CNNs) for image processing can enable the creation of applications that understand and generate text based on visual inputs. This integration requires sophisticated techniques to ensure seamless interaction between different types of models and data.
Applications and Use Cases
Healthcare
In healthcare, multimodal applications can enhance diagnostic accuracy by combining textual and visual data. For example, a diagnostic tool could analyze medical images alongside patient records, leveraging LLMs to interpret textual information and CNNs to process visual data. This holistic approach can lead to more accurate diagnoses, quick patient care, and personalized treatment plans.
Entertainment
The entertainment industry can benefit from AI-generated content that combines text, images, and audio to create immersive experiences. For instance, an AI system could generate interactive stories that adapt based on user inputs, incorporating visual and audio elements to enhance engagement. LLMs can generate narrative text, while other models handle the visual and auditory components, creating a cohesive and engaging experience.
Edtech
Intelligent tutoring systems in the edtech sector can leverage multimodal AI to provide a more comprehensive learning experience. These systems can use text, speech, and visual aids to deliver personalized instruction and feedback. For example, an intelligent tutor might use LLMs to generate explanations and answers, while utilizing speech recognition to interpret student questions and visual aids to illustrate concepts.
Customer Service
This is one area where we have seen the increasing use of LLMs. Virtual assistants in customer service can benefit from multimodal AI by understanding and responding to text and voice inputs while analyzing visual data. This enables more intuitive and effective interactions with customers.
For example, a virtual assistant might use LLMs to generate text responses, speech-to-text models to process voice inputs, and image recognition models to analyze visual data, providing a comprehensive and seamless customer service experience.
Conclusion
By combining the strengths of LLMs with other AI models, we can develop applications that process and understand multiple data types, leading to more accurate, context-aware, and engaging interactions. As technology continues to evolve, the possibilities for multimodal AI are vast, promising significant advancements across various domains, from healthcare and entertainment to education and customer service. To know more about our Gen AI services, contact us and see how we can fuel your next project.