Enhancing Quality Control in Manufacturing with IoT-Driven Asset Management: A Technical Perspective
- September 25, 2024
- Posted by: Sanket Pathak
- Category: Industrial Asset Management
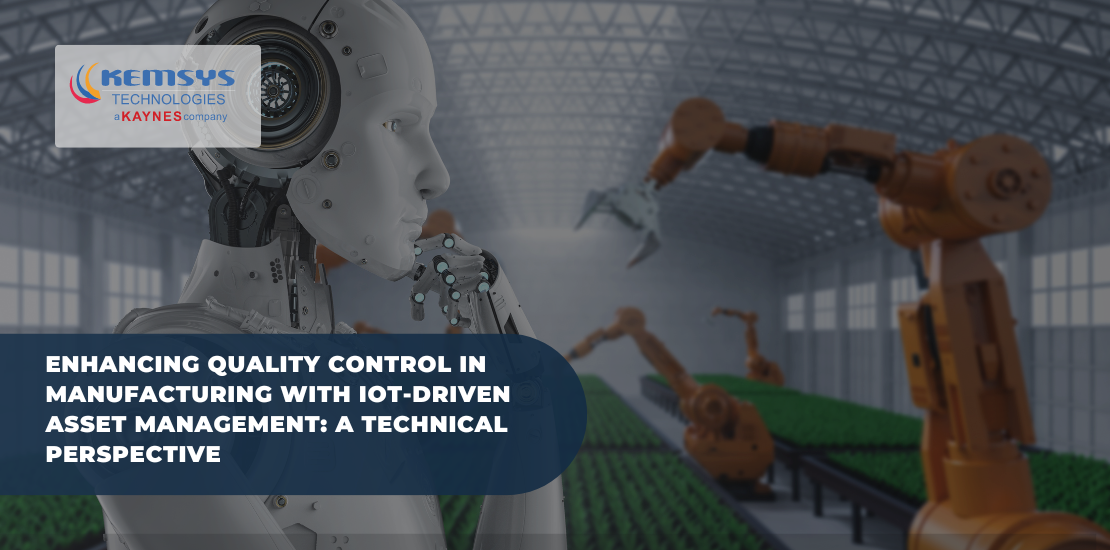
The convergence of IoT-enabled asset management systems and advanced manufacturing methodologies has revolutionized quality control frameworks, delivering granular, real-time insights into every facet of the production lifecycle.
By harnessing the power of IoT-based systems, manufacturers are now able to implement highly sophisticated monitoring systems, ensuring reliable processes and deploying predictive analytics to preempt quality issues and deliver quality products.
Advanced Product Quality Monitoring with IoT-Based Asset Management
Data Acquisition Layer: Precision IoT Sensors and Cyber-Physical Systems
The deployment of IoT sensors within manufacturing lines forms the backbone of real-time data acquisition systems. These sensors help in gathering metrics and insights to enable real-time monitoring:
- Optical Metrology Systems: High-resolution optical sensors and laser triangulation systems provide micron-level accuracy in dimensional measurements, crucial for ensuring adherence to tight tolerances.
- Thermocouple Arrays: In thermally-sensitive production lines, multi-point thermocouples facilitate precise temperature control, ensuring process parameters remain within critical operational windows.
- Multispectral Vision Systems: Advanced imaging technologies, spanning visible to near-infrared spectrums, enable the detection of chromatic deviations and surface defects with sub-pixel accuracy. These systems are particularly effective for high-gloss or reflective surfaces.
- Automated Inline Inspection Systems: Leveraging robotic arms and automated vision systems, inline inspection ensures real-time validation of products, reducing manual interventions and enhancing throughput without sacrificing accuracy.
Related Content: Enhancing Incident Reporting with IoT: Streamlining Asset Loss and Theft Management
Real-Time Edge Analytics for Low Latency Feedback Loops
The vast amount of data generated by sensors needs real-time processing to ensure low-latency feedback within the manufacturing process. This is where edge computing comes into play, as it addresses the processing of data at the edge, enabling faster decision-making.
By analyzing sensor data directly on the manufacturing floor, edge analytics minimizes the need to send data to the cloud, reducing latency and providing real-time feedback on key quality metrics. This is essential for maintaining smooth operations, as any delays in detecting quality issues can lead to costly production errors.
Edge nodes, which aggregate and analyze data locally, are central to real-time edge analytics. These nodes employ machine learning algorithms like decision trees and random forests to assess sensor data and identify deviations from established quality thresholds.
For instance, an edge node monitoring temperature during a heat treatment process can trigger instant alerts if values fall outside the acceptable range, enabling on-the-spot corrective actions. This ensures defective products don’t progress through the production line.
Parallel data processing is another benefit of edge analytics. While critical data is processed locally for immediate decisions, it is also sent to the cloud for further analysis and storage. This dual approach allows manufacturers to combine real-time insights with historical data analysis, identifying recurring issues that may not be evident from real-time data alone.
Automated Quality Alerts and System Responses
Automation is key in maintaining quality control with minimal human intervention. In an IoT-driven environment, where thresholds for parameters such as temperature and pressure are predefined, continuous monitoring is necessary. When deviations are detected, automated alerts—via emails, SMS, or system notifications—are triggered, ensuring immediate attention to potential issues. Programmable Logic Controllers (PLCs) can automatically halt the production process or reroute defective products for reinspection when such deviations occur. This automated system ensures consistent product quality while minimizing human error and downtime.
Dynamic quality gates, placed at various production stages, also help maintain high standards. These gates act as checkpoints, ensuring only products meeting all quality criteria proceed to the next stage. If a product fails to meet the standards, it is flagged or rejected, reducing the chances of defective items reaching customers.
Process Consistency with IoT-Based Control Systems
IoT-based asset management systems enforce process consistency by using digital Standard Operating Procedures (SOPs). These SOPs are integrated into workflow automation engines, monitoring every step of the production process to ensure adherence to operational standards.
Real-time data streams are continuously compared to these SOPs, and any deviations are flagged for correction, reducing process variability and ensuring consistent quality across production lines. Machine learning models also drive adaptive optimization by analyzing historical data to fine-tune processes. For example, if temperature fluctuations are identified as a defect source, the system can automatically adjust heating parameters to ensure consistent quality.
Advanced Defect Detection and Root Cause Analysis
Machine vision systems, powered by deep learning algorithms, have transformed defect detection. These systems use high-resolution cameras and advanced image processing to inspect products for defects like cracks or misalignments.
Convolutional Neural Networks (CNNs) can analyze images, identifying even the smallest defects with high accuracy. As more data is collected, the system can be further improved through unsupervised learning, continuously enhancing its ability to detect subtle deviations. This reduces reliance on manual inspections while maintaining high-quality standards.
Once a defect is detected, root cause analysis helps identify underlying causes. By correlating defect data with manufacturing conditions using multivariate time-series analysis, manufacturers can identify relationships between process parameters and defects. Causal inference models can provide further insights, helping address specific issues and reduce future defects. Targeted improvements based on root cause analysis enhance product quality and at the same time reduce the likelihood of recurring issues.
Preventive and Predictive Maintenance Systems
Predictive maintenance is a critical feature of IoT-driven asset management, enabling manufacturers to anticipate equipment failures before they impact production. Vibration and acoustic sensors continuously monitor equipment health, and machine learning algorithms analyze the data to predict potential failures. Automated maintenance work orders are generated when issues are detected, ensuring equipment is repaired before problems escalate. This minimizes downtime and prevents quality degradation, helping manufacturers maintain consistent production levels.
Advanced Analytics and Reporting for Continuous Improvement
Finally, IoT systems offer advanced reporting capabilities, allowing manufacturers to track key quality metrics. Customizable reports highlight trends in defect rates and equipment effectiveness, offering insights for continuous improvement.
Cloud-based storage enables long-term data analysis, helping manufacturers benchmark performance and understand factors that impact the quality of the products over time. By leveraging these advanced analytics, manufacturers can optimize processes and maintain a competitive edge.
Conclusion
The integration of IoT-driven asset management systems in manufacturing offers transformative benefits for maintaining product quality and operational efficiency. From real-time edge analytics to automated alerts and predictive maintenance, these technologies enable manufacturers to make data-driven decisions and respond proactively to potential issues.
To learn more about our IoT-based asset management services, get in touch with us.