LLMs in Conversational AI and Chatbots: Elevating User Interactions
- August 16, 2024
- Posted by: Anand Borad
- Category: Artificial Intelligence
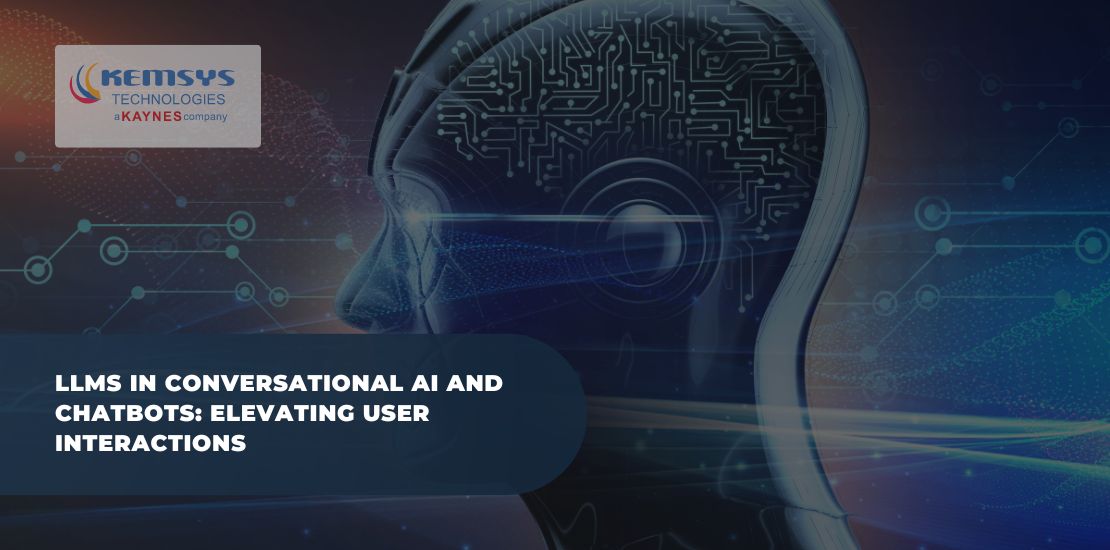
We are all familiar with chatbots, especially when it comes to customer service on various online platforms. Conversational AI and chatbots have transformed the way businesses engage with their customers, offering instant support, personalized experiences, and round-the-clock service.
While traditional rule-based chatbots have been instrumental in automating customer interactions, the advent of Large Language Models (LLMs) has taken conversational AI to new heights. These advanced models, powered by deep learning, have significantly enhanced the ability of chatbots to understand, respond, and adapt to user inputs, making interactions more human-like, engaging, and fruitful at the same time.
The Evolution: From Rule-based bots to Conversational AI
Chatbots have evolved remarkably from their early days. Initially, the rule-based chatbots were only limited to their predefined scripts and lacked the flexibility and intelligence to handle complex queries. As technology progressed, AI-driven conversational agents emerged, offering more dynamic and context-based interactions.
However, even these systems had their set of limitations, particularly in understanding nuanced language and often maintaining the conversational context. The introduction of LLMs marked a significant shift, addressing many of these challenges and enabling a new era of conversational AI that is more intuitive, responsive, and capable of delivering richer user experiences.
According to MarketsandMarkets, the conversational AI market is projected to grow from USD 13.2 billion in 2024 to USD 49.9 billion by 2030, at a compound annual rate (CAGR) of 24.9% during the forecast period.
The market has experienced this high growth due to emerging technologies like Generative AI. With the integration of technologies such as computer vision and voice recognition, conversational AI is only going to get better.
Related Content: Harnessing the Power of Large Language Models for Multimodal AI
Enhancing Chatbot Capabilities with LLMs
Natural Language Understanding (NLU)
LLMs have significantly improved the Natural Language Understanding (NLU) capabilities of chatbots. By training on diverse datasets, LLMs can comprehend a wide range of languages, dialects, and expressions, allowing chatbots to interpret user input with greater accuracy.
For instance, LLMs like GPT-4 have been fine-tuned to recognize and understand the subtle nuances in human language, enhancing the chatbot’s ability to engage in meaningful conversations and generate accurate results.
Contextual Awareness
Maintaining context over extended conversations is crucial for delivering seamless user experiences. LLMs excel at retaining and managing context, allowing chatbots to reference earlier parts of a conversation and respond appropriately. This contextual awareness not only improves the flow of dialogue but also increases user satisfaction by making interactions feel more natural and coherent. Contextual awareness in an LLM also helps in generating more accurate results.
Response Generation
LLMs have drastically improved response generation in chatbots, enabling them to produce more coherent and human-like replies. Through techniques such as fine-tuning, LLMs can generate responses that are relevant, accurate, and aligned with the user’s intent. This advancement is evident in customer service applications, where chatbots can now handle complex queries with a level of sophistication that was previously unattainable.
Personalization and Adaptability
One of the key strengths of LLMs lies in its ability to personalize interactions based on user preferences and history. By analyzing past interactions, LLMs can tailor responses to individual users, making the conversation more relevant and engaging. Additionally, adaptive learning mechanisms allow chatbots to evolve over time, refining their behavior to better meet user expectations.
Multilingual Support
In today’s globalized world, multilingual support is essential for reaching a diverse user base. LLMs enhance multilingual capabilities by training on multilingual datasets, enabling chatbots to support multiple languages seamlessly.
Companies like Google and Microsoft have successfully implemented LLMs to develop chatbots that cater to users across different linguistic backgrounds, ensuring consistent and high-quality interactions.
Challenges and Considerations
While LLMs offer numerous benefits, there are challenges that must be addressed. Let’s take a look at some of them.
Data Privacy and Security
One of the frequently asked questions has always been about data privacy. Ensuring user data protection and compliance with privacy regulations is extremely important. As LLMs process vast amounts of data, safeguarding this information and maintaining transparency about its use are critical.
To address data privacy concerns, it’s essential to implement robust encryption methods for data in transit and at rest. Regular audits and compliance checks with relevant data protection regulations, like GDPR or CCPA, can help ensure that user data is handled responsibly.
Bias and Fairness
LLMs are susceptible to biases present in the data they are trained on. It is essential to implement strategies to mitigate these biases and ensure that chatbot interactions are fair and unbiased.
To mitigate bias, businesses should prioritize diverse and representative data sets during the training phase. Regular audits of the model’s outputs for biases and implementing fairness checks are essential. Employing techniques like re-sampling, re-weighting, or adversarial training can also help in reducing bias.
Scalability and Performance
The computational resources required to deploy LLMs at scale can be significant. Optimizing performance while managing costs is a key consideration for businesses looking to leverage these models.
While deploying LLMs, it’s important to always assess the computational requirements and invest in scalable infrastructure, such as cloud-based solutions, that can handle the load. Optimization techniques, like model compression, quantization, and efficient hardware utilization, can reduce the computational footprint. Implementing load balancing and auto-scaling can ensure consistent performance even during peak usage.
User Trust and Acceptance
Building and maintaining user trust in AI-driven interactions is crucial. Ensuring that chatbots behave ethically and transparently will be vital in fostering user acceptance. These factors play a vital role in making the customer experience better.
Providing clear information about how the chatbot operates, including its limitations and the extent of its capabilities, can help set realistic expectations. Incorporating feedback loops where users can report issues or biases and receive responses can also foster trust.
Conclusion
LLMs have transformed conversational AI to enable chatbots to deliver more human-like, engaging, and effective interactions. As these models continue to evolve, their integration into various applications will further enhance user experiences, driving the future of customer engagement and support. For businesses looking to stay ahead of the curve, embracing LLMs in their chatbot strategies is not just an option—it’s a necessity for now and for the future.
To know how Kemsys Technologies can help you build chatbots that offer exceptional user experience, get in touch with our experts.